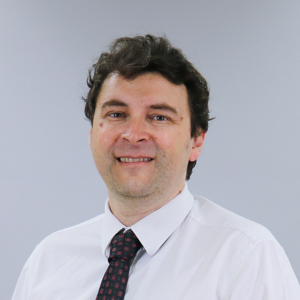

Xavier Roger Gilbert BRESSON
Associate ProfessorXavier Bresson is an Associate Professor in the Department of Computer Science at the National University of Singapore (NUS). His research focuses on Graph Deep Learning, a new framework that combines graph theory and neural networks to tackle complex data domains. In 2017, he received the USD 2M NRF Fellowship, the largest individual grant in Singapore, to develop this new framework. He was also awarded several research grants in the U.S. and Hong Kong. He co-authored one of the most cited works in this domain (10th most cited paper at NeurIPS) and has significantly contributed to mature these emerging techniques. He has organized several conferences, workshops and tutorials on graph deep learning such as the 2022 conference on “Learning on Graphs”, the IPAM'23 workshops on "Learning and Emergence in Molecular Systems", the IPAM'23'21 workshops on "Deep Learning and Combinatorial Optimization", the MLSys'21 workshop on "Graph Neural Networks and Systems", the IPAM'19 and IPAM'18 workshops on "New Deep Learning Techniques", and the NeurIPS'17, CVPR'17 and SIAM'18 tutorials on "Geometric Deep Learning on Graphs and Manifolds". He has been a regular invited speaker at universities and companies to share his work. He has also been a speaker at the NeurIPS'22, KDD'21, AAAI'21 and ICML'20 workshops on "Graph Representation Learning", and the ICLR'20 workshop on "Deep Neural Models and Differential Equations". He has taught undergraduate and graduate courses on Deep Learning and Graph Neural Networks since 2014. Online profiles: Twitter (8,000+ followers): shorturl.at/ilnB1, GScholar (15,000+ citations/60+ articles): shorturl.at/iuy59, GitHub (2,500+ stars): shorturl.at/MQWY8, YouTube (70,000+ views): shorturl.at/eg279, LinkedIn: shorturl.at/mvzMW
RESEARCH AREAS
RESEARCH INTERESTS
Machine Learning
Graph Neural Networks
Sparse Convex Optimization
Spectral Graph Theory
RESEARCH PROJECTS
RESEARCH GROUPS
TEACHING INNOVATIONS
SELECTED PUBLICATIONS
AWARDS & HONOURS
MODULES TAUGHT