Like so many parts along the Californian coast, Honda Point is breathtakingly beautiful. People go to visit, but when they do, it’s not for the views.
Rather, they go to remember one of the darkest days in U.S. Naval history, when seven destroyers ran aground and twenty-three sailors perished. Lieutenant Commander Donald T. Hunter, who was in charge of navigating the ships from San Francisco to San Diego that day, relied primarily on the centuries-old technique of dead reckoning. A more accurate method called radio direction-finding (RFD) had been invented two years earlier, but Hunter was mistrustful of the new technology — a decision that would ultimately prove fatal.
The Honda Point tragedy happened close to a century ago, but it still holds a timely lesson: humans and machines need to trust one another. The message is ever more salient today as machines — both software systems and physical robots — become a ubiquitous part of our daily lives. Everywhere we look, our tech companions are being afforded greater autonomy: self-driving cars are being trained to make life-and-death decisions, machine learning algorithms make a first pass at job seekers, artificial intelligence programmes write news stories for reporters, and so on. If we are to live and work alongside machines, mutual trust is key.
“There is human trusting robots and there is also robots trusting humans — it’s a two-way street,” says NUS Computing assistant professor Harold Soh, who first became fascinated with the notion of trust in robots more than a decade ago during his PhD. Today, Soh runs the Collaborative Learning and Adaptive Robots (CLeAR) group, which studies human-AI/robot collaboration.
“We are working towards something called mutual trust calibration,” says Soh. “What we want to do is to get robots to understand when to trust humans, and for human beings to understand when to trust robots.”
“We believe that well-calibrated trust leads to a beneficial long-term collaboration between humans and robots,” he says.
To that end, Soh and his team are trying to mathematically model the notion of trust using techniques from machine learning and AI. They also run social experiments with human subjects in order to validate their theories — a dual approach that differentiates the CLeAR group from others in the field.
A rich mental model
Trust, for all its pervasiveness in our lives (underpinning relationships, allowing us to function in society by trusting in institutions, the government, etc.), can be a tricky concept to define. “It’s something that is internal to human beings, and you don’t actually see trust,” says Soh. “Trust does affect behaviour, though, and we can infer how much your trust is depending on how you behave.”
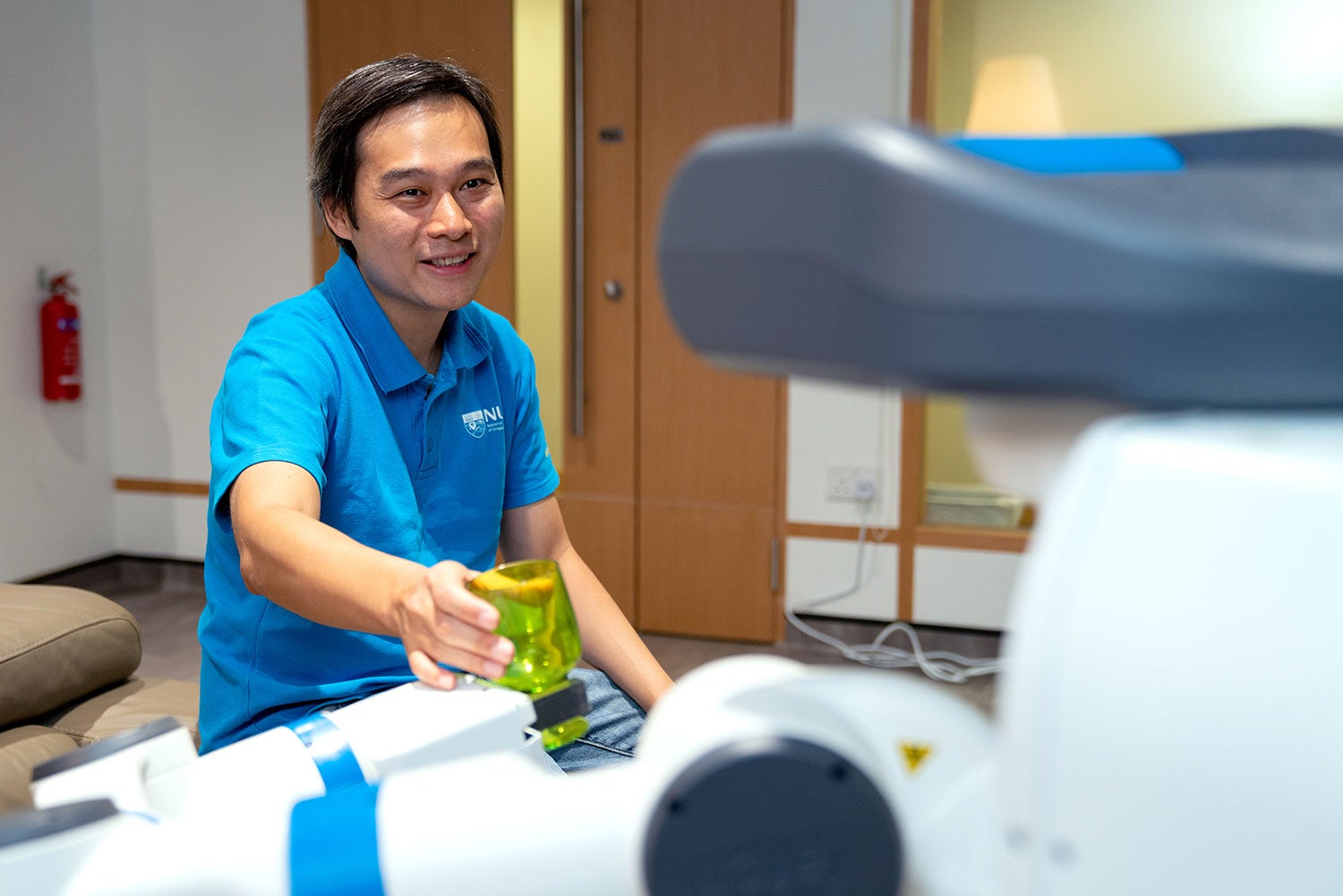
Soh’s team has conducted experiments centred around one fundamental question: when do humans decide whether to trust a robot or not? It turns out that there are two main factors people consider: capability and intention.
“Before people decide to trust the robot, they check whether the robot is physically capable of achieving its goal, and then check whether it has similar intentions,” explains Soh. In one experiment, they recruited 400 participants to play an online game involving fire-fighting drones dropping water over various hotspots.
People, they realised, would decide whether to take-over control from the drone or let it operate autonomously, by estimating how capable it was (Could it find the spots that were on fire? Would it succeed in firefighting given the prevailing weather conditions?) and what its “intentions” were (What kind of risks would it take to clear the hotspots?).
More importantly, however, the researchers recognised that human-robot trust relationships are much more complex, involving factors beyond evaluations of robot capability and intention. “Trust is a rich mental multi-dimensional construct,” says Soh.
His team explored these other influences in a separate paper published last year. Surveying a group of more than 30 participants, the researchers found that a person tended to trust a robot more, at least at the outset, if they had prior familiarity with robots or if they had played video games. Gender and computer usage were found to have no impact on initial trust levels.
Trust in robots also depends on the context and the task that the robot is trying to perform. The team found that human trust “transfers” across similar tasks. For example, participants were more likely to trust a robot to pick up a can of chips if they had seen the robot successfully perform a similar task in the past, such as picking up an apple. But this trust doesn’t carry over as much to dissimilar tasks such as navigating safely in a room. Using these insights, they team fashioned a new kind of trust model using a human’s “psychological task space,” which represents how similar or different tasks are from a human’s perspective.
Robots that judge
When it comes to trust, having the right amount is key, says Soh. Too much trust and you end up with situations such as drivers falling asleep in their Teslas with the Autopilot on; too little and tragedies like Honda Point occur.
With this in mind, Soh has been searching for ways to help robots gauge a human’s trust in them. “When a robot understands trust, it can modify its decision-making appropriately, which leads to better collaboration outcomes with people,” he says.

In 2018, Soh and Professor David Hsu (also at NUS Computing), together with collaborators from the University of Southern California and University of Washington, built a computational model for integrating human trust in robot decision-making. They demonstrated the model in an experiment where participants had to work together with a robotic arm to clear objects, ranging from plastic bottles to a wine glass, off a table.
In one of the experiments, the robot’s first action was to gauge the participant’s level of trust in it, by attempting to pick up a medium-risk object (a fish can). If the person intervened to stop the robot, it signalled a low level of trust. The robot would then proceed by successfully picking up the plastic bottles, before moving onto the wine glass.
Conversely, if the person allowed the robot to pick up the fish can, it indicated a high trust level. The robot could then respond to by intentionally failing to grasp the plastic bottles — a signal for the user to be wary when it came to the fragile wine glass. “However, intentional failures can have costs and be viewed as deceptive, and should be well thought-out before actual use,” says Soh.
“The robot should monitor human trust and influence it so that it matches the system capabilities,” write the authors in their paper. That’s important because robots may fail, and they need to communicate their capabilities to the people using them.
“It’s about calibrating human trust to improve human-robot team performance over the long run,” says Soh. “Ultimately, we think that if you model trust well, it will lead to positive outcomes where humans and robots collaborate effectively to solve problems.”
Paper:
Robot Capability and Intention in Trust-based Decisions across Tasks
Multi-Task Trust Transfer for Human-Robot Interaction