Stéphane Bressan and Christian Miniatura grew up in rival neighbourhoods of the naval garrison town of Toulon in southern France. They went to the same high school and the same college only a few years apart, but never were acquainted until 2006 when they were both working halfway across the world, at the National University of Singapore. Miniatura and Bressan became fast friends, meeting regularly to “put the world to rights” over French food and wine.
“One of our favourite debates was whether artificial intelligence can be useful to quantum physics,” says Bressan, an associate professor at the School of Computing. He was convinced that AI could lend a helping hand in solving some of physics’ longstanding problems. But Miniatura, a quantum physicist by training and the director of the Franco-Singaporean physics laboratory MajuLab, remained perplexed albeit intrigued at the possibility.
“You don’t always speak the same language when you are doing science in different branches, writing about different topics,” says Bressan.
But that all changed in February 2017, when two computational physicists, Giuseppe Carleo and Matthias Troyer, published a paper suggesting how the fields of computer science and quantum physics could be brought together.
“For a long time, Christian and I could not understand each other,” recalls Bressan. “But when Carleo and Troyer built this bridge with their paper, then it became all clear to us.”
Miniatura adds: “All of a sudden, they gave substance to our past discussions and I could clearly grasp what Stéphane was saying to me, what kind of things we could be doing.”
The “curse” of multiple dimensions
The 2017 paper proved seminal on a wide scale, but also for Bressan and Miniatura, who began collaborating soon after. Specifically, the paper showed how a machine learning tool, called neural-network quantum states, could be used to study a notoriously hard problem in physics: the quantum many-body problem.
The problem pertains to systems comprised of a large number of particles that obey the laws of quantum physics. Physicists describe these a systems by a wave function, which is a fundamental object that allows for them to compute the likelihood of the possible states the system can be in, as represented by the positions, velocities, spin, and other characteristics of its component particles.
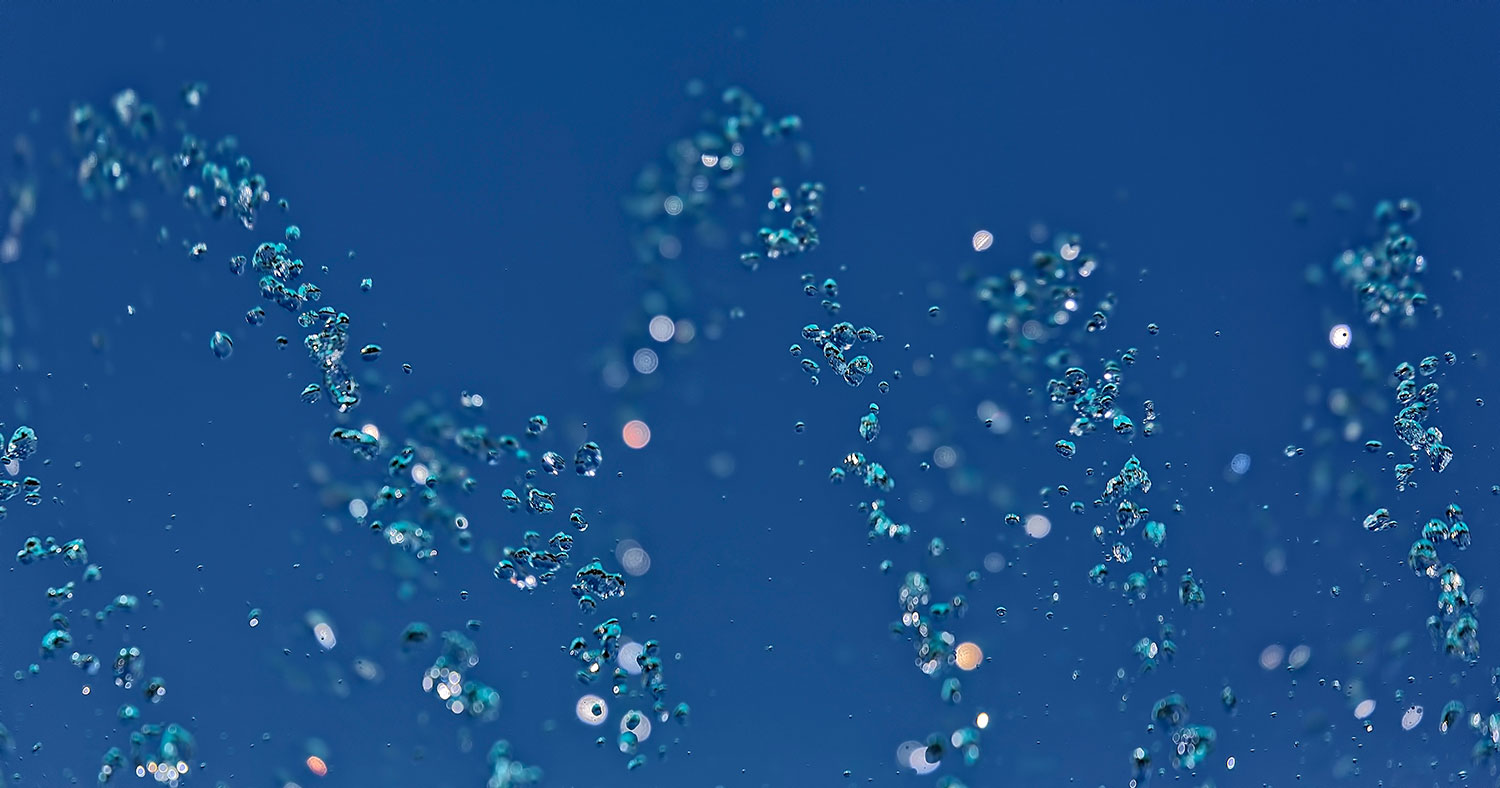
The challenge that has stumped physicists for decades, says Miniatura, is that the number of possible states of a system “becomes exponentially large with the number of particles, rapidly outperforming the capabilities and resources of the best computers to date”. Describing and understanding the basic properties of such quantum macroscopic systems is thus a formidable task that ranks among the most computationally intensive challenges in science.
Drawing inspiration from Carleo and Troyer’s 2017 paper, Bressan and Miniatura, alongside other collaborators, decided to explore whether the machine learning tool proposed in the paper could be both scaled up and applied at a faster rate to large-sized quantum systems.
“The simplest problem we can look at for a quantum neural network is to calculate the minimum energy state it can settle in,” explains Bressan.
Finding this minimum energy is to optimise things, he says. “Therefore, what we are trying to do is solve an optimisation problem, which is very difficult because in order to compute the actual values, you need to compute them at the limits where there are infinitely many particles,” he says. “The size of the space you must explore to find a solution has many dimensions.”
Transfer learning: Learning from before without supervision
Many AI systems learn to perform a task by being shown examples — something that is referred to as supervised learning. In contrast, the neural networks Bressan refers to learn by minimising the energy — something that is referred to as unsupervised learning.
A neural network previously trained to recognise pictures of dogs, for example, can be retrained without too much difficulty to be able to spot cats as well, because the two “are both furry animals, have four legs, two pointy ears, etc.,” says Bressan, describing an approach called transfer learning.
“The idea of transfer learning is that you use a model designed for one task and you re-tune it for another task, which hopefully saves you training time,” says Bressan. He and his collaborators believed that transfer learning from one network to another could help speed up and scale up the approach to solving the dimensionality challenge of the quantum many-body problem.
“The idea we explored is how to take an existing network that we have trained for 10 particles in a certain state and transfer it, for example, to a network of 20 particles, from a smaller system to a bigger system, in a different but nearby state” he says.
Through various tests, they managed to prove that machine learning can indeed be used to accelerate, scale up and solve problems that have an ever increasing number of particles.
Neural networks allow you to scale up for one simple reason, says Bressan. Their architecture makes them highly prone to parallelism, which means that the computation can be done by many computing units in parallel, for example on graphics processing units, or GPUs — hardware that is cheap and widely available (fueled partly by gamers who wanted excellent graphics, GPUs are found in mobile phones, personal computers, workstations, and game consoles).
“In the past, physicists had to build from scratch using whatever hardware was available and programme everything on their own,” says Bressan. “Now, with neural networks, we have generic software platforms and various hardware available in the form of GPUs and supercomputing infrastructures, so we can develop things much faster and get competitive results.”
Getting critical
Bressan and his collaborators then tested the machine learning tool on a specific quantum many-body problem: how to find the critical point where a system switches between two completely different states, for example when a liquid transitions to a gas.
Starting with a small-sized system that was in a state for which they knew how to construct a network, the researchers then explored other states by gradually transferring what had been learned until they identified the system’s critical point. They then transferred that knowledge once more to find the critical point of increasingly larger systems. Experiments showed that their approach proved more effective and efficient than other approaches to which they compared.
Towards science-making machines
The team now wants to examine more challenging problems such as the different possible states of matter of these systems and the transitions between them, as well as systems with few strongly interacting particles.
More generally speaking, they want to keep exploring how AI can help tackle problems in physics and other fields of science.
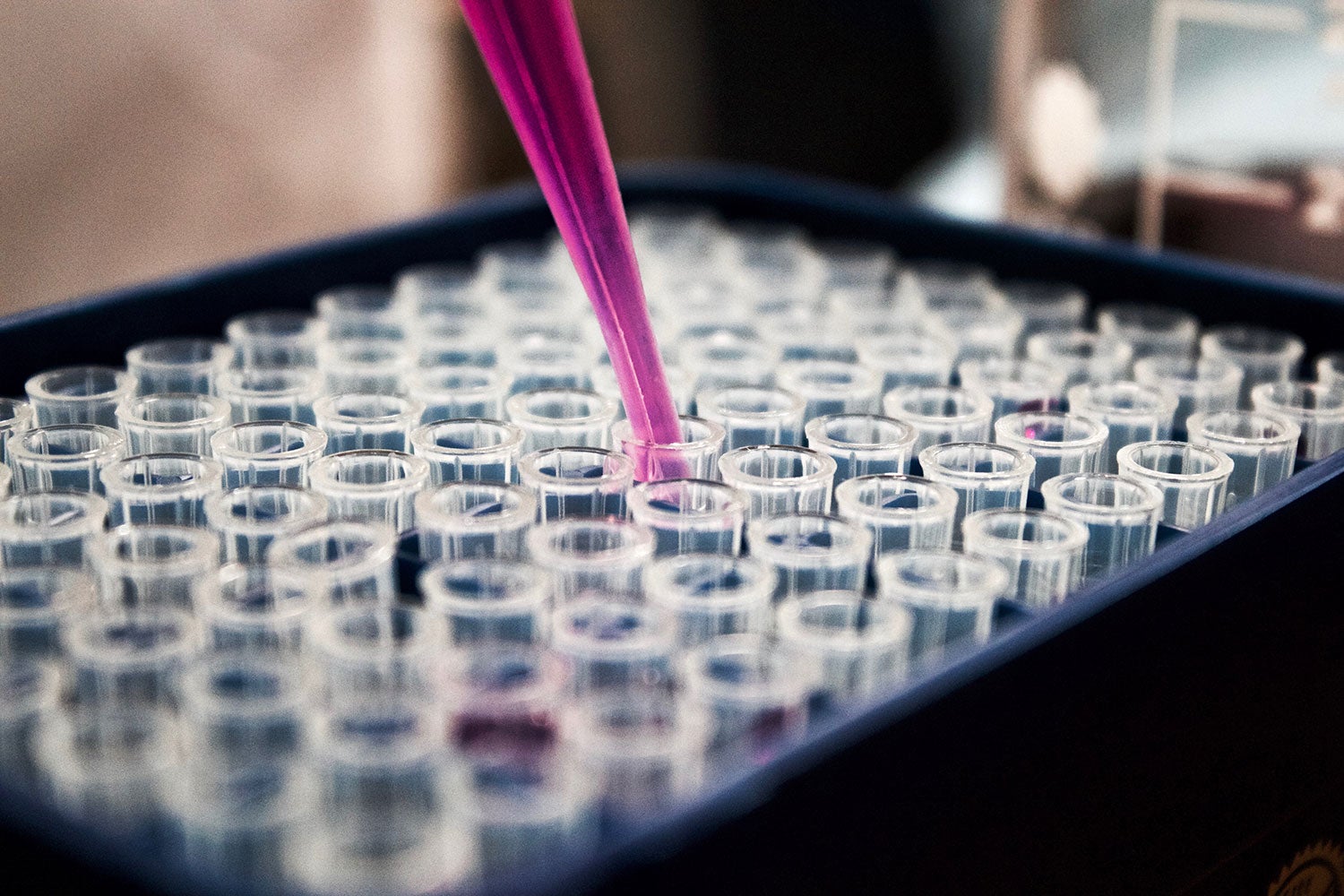
“We have seen the success of AI in various popular domains and many everyday applications, like video and image processing, natural language translation, and the games of Go and chess,” says Bressan. “But now is the time when we can start thinking about its impacts on fundamental science.”
“Computers are indispensable tools for scientists. AI can now help us make new discoveries,” he says. “But can AI actually discover new scientific concepts? That is the big question.”
Bressan and Miniatura both say: “We don’t know the answer but we are exploring this question.”
Papers:
Finding Quantum Critical Points with Neural-Network Quantum States
Transfer learning for scalability of neural-network quantum states