Imagine if Amazon Alexa could recommend a tub of ice cream or Siri could play a cheerful song if they hear sadness in your voice. AI voice recognition can now recognise emotions with very high accuracy. Yet it is not correct all the time, and this begs the question of how it make its decisions.
The quest to unscramble these questions belong to the realm of Explainable AI (XAI), which seeks to explain the results produced by machine learning algorithms. With AI becoming ubiquitous in our everyday lives, XAI is a nascent but growing field. However, it has much room for improvement, says Brian Lim, an assistant professor at NUS Computing who has spent more than a decade researching the topic. “A lot of XAI techniques are difficult to understand because they’re far too technical.”
“We need to make the explanations more meaningful to people and more relatable to the knowledge that we are familiar with. We must make XAI more human-centric,” says Lim.
It’s a goal that only a handful of research groups around the world are currently striving towards, and Lim’s lab, the NUS Ubicomp Lab, is “one of the rare ones that can actually define and develop human-like explanations,” he says.
Getting AI to think like a human
In order to obtain such human-like explanations, Lim and his PhD student Wencan Zhang studied theories from cognitive psychology and learned about the human perceptual process.
To understand the process, consider how our brains recognise an animal in a picture. The reasoning steps include: selecting salient parts of the scene (for instance the animal’s attractive ears); organising them into semantic cues (recognising that they are ears); recalling various examples of similar cues (pointy, floppy ears, etc.), and comparing them to draw a conclusion of what the overall concept or object is (that the ears, eyes, and mouth are more similar to that of cats than of dogs). By weaving all of these together, our brains can come to a conclusion and work out what it’s seeing (a kitten!).
With these steps, Lim realised that they could represent and justify several recent popular techniques in XAI — namely, saliency, counterfactual, and contrastive explanations. He and Zhang thus proposed the XAI Perceptual Processing Framework. He explains: “We have identified a framework to unify various explanations to support multi-faceted understanding.”
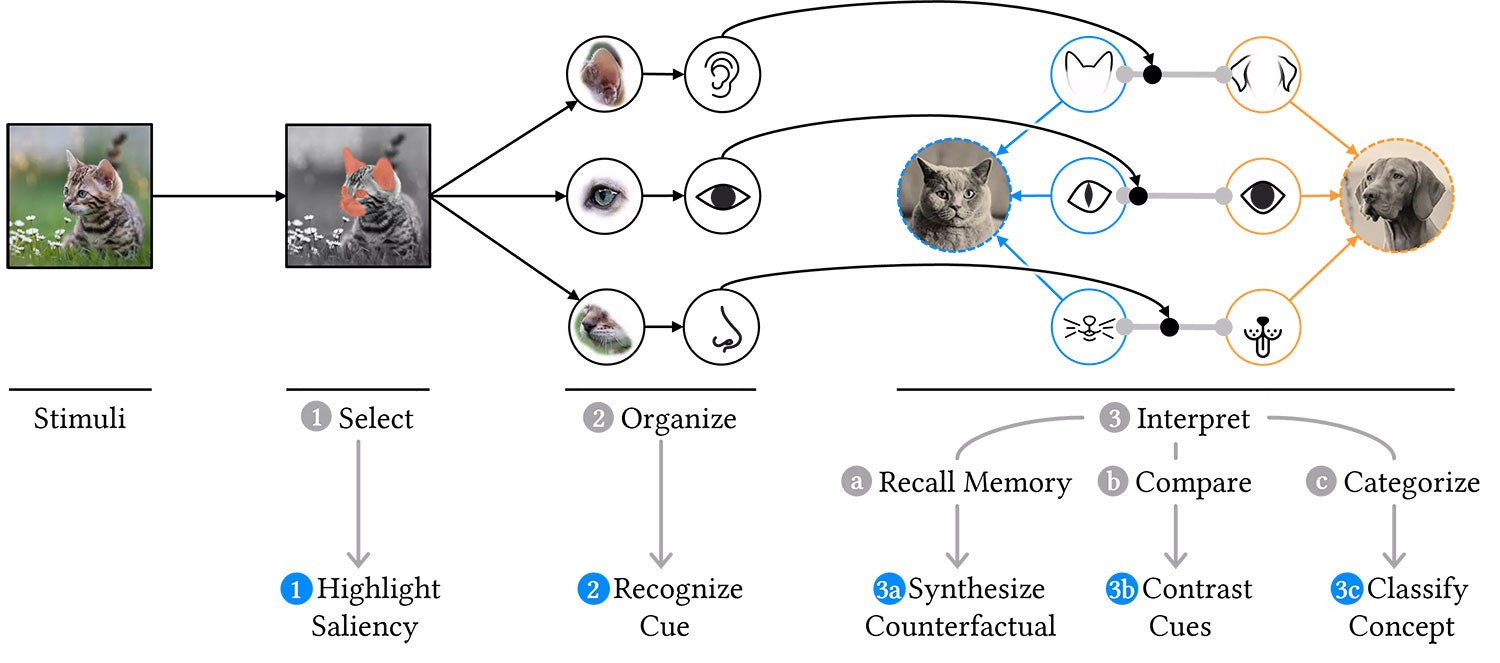
The pair then implemented the framework as RexNet (Relatable Explanation Network) — a deep learning model with modular interpretable components leveraging state-of-the-art AI techniques.
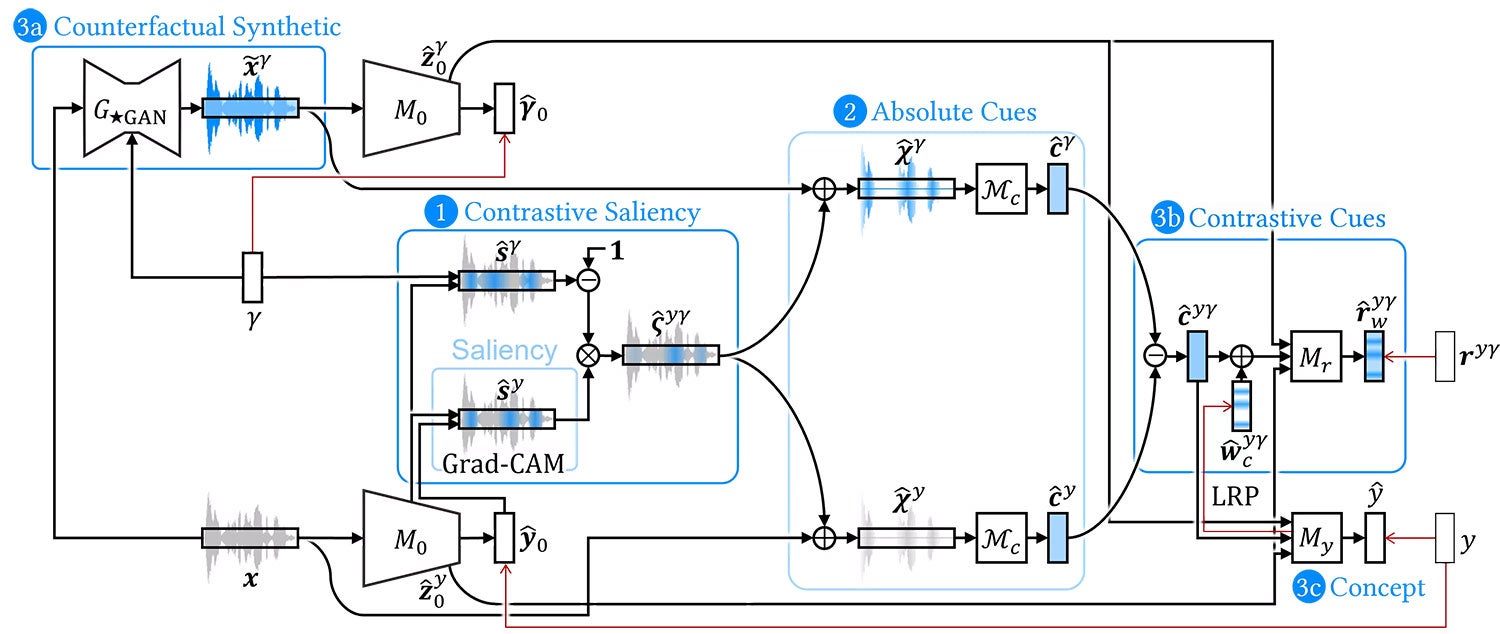
“Integrated under one roof, the multiple explanations are coherent and consistent with one another,” says Lim. “And since RexNet is reasoning more intuitively and relatably, we get the bonus that it makes predictions more accurately too!”
Relatable explanations for vocal emotions
With RexNet, the AI can make more relatable and rounded explanations of various predictions, including explaining the emotion in voice. On hearing a person’s speech, the system may predict the voice as “surprised” and provide several explanations to help the user to understand by relating to other emotions, relating to various audio cues, and relating to salient moments.
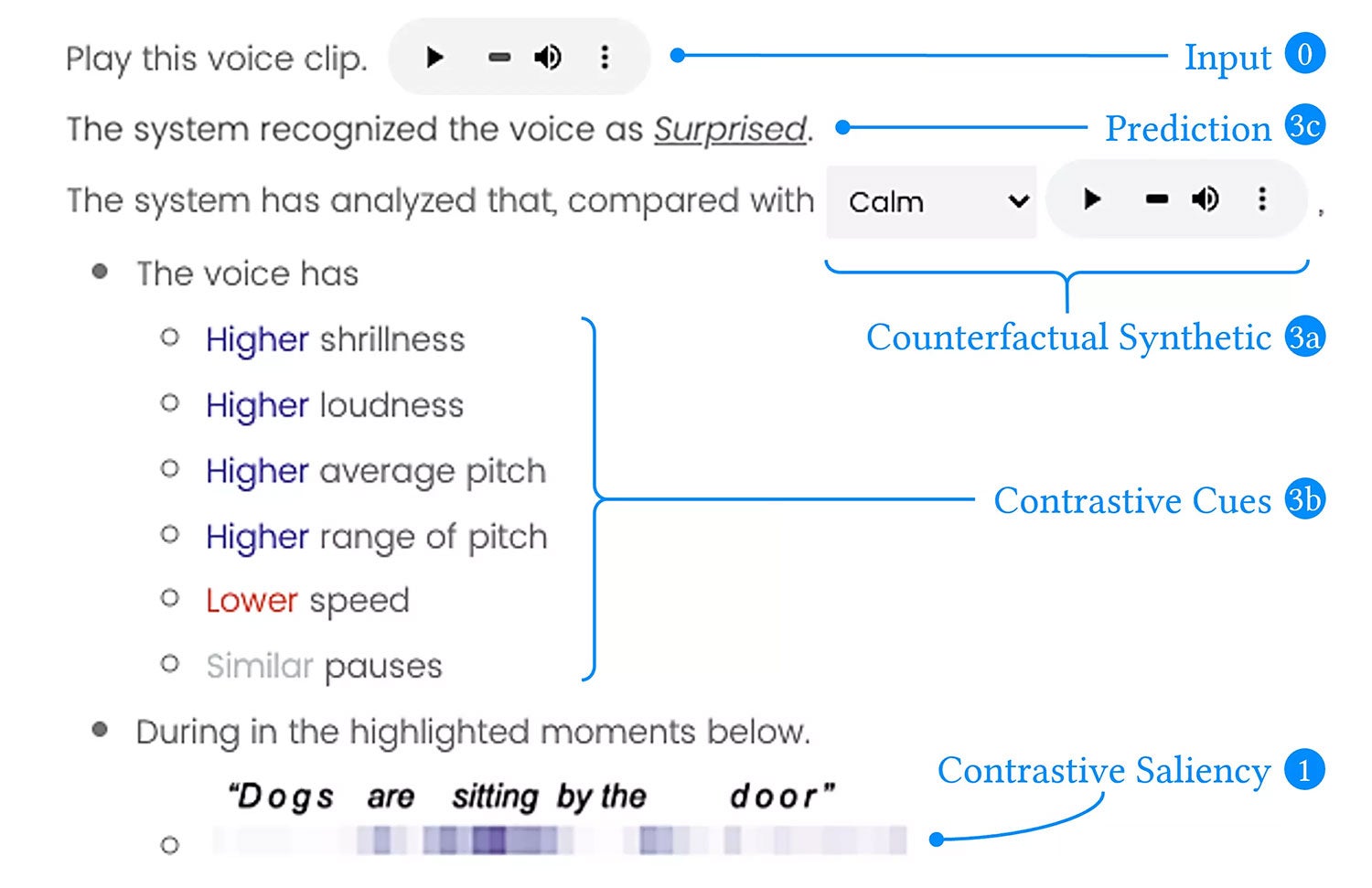
To test the effectiveness of their new system, the pair ran it through a gamut of tests. They first conducted a modelling study which demonstrated that RexNet can indeed predict vocal emotions more accurately when compared with state-of-the-art prediction techniques (79.5% versus 75.7%) and provide faithful explanations.
This was followed by a qualitative user study to examine how users make use of the various types of explanations, before a final — much larger — quantitative user study involving 175 participants. The aim of the last study was to evaluate how useful participants found each type of explanation when trying to infer vocal emotions.
The findings revealed that participants benefited the most from counterfactuals and cues, but saliency heatmaps were still considered technical and thus less useful. “There is a benefit to combining explanations, but you need to be careful in fine tuning it,” explains Lim.
In all, the more a machine thinks like a human, the wiser it is, he says. “Not only is it more interpretable, it actually performs better.” And importantly, the more human-centric XAI is, the more people tend to trust it.
Lim and Zhang will present their findings at the prestigious conference on Human Factors in Computing Systems (CHI) on May 3 this year. The paper they submitted was awarded the prize for Best Paper — a recognition granted to the top 1% of submissions.
Next up, they plan to include additional types of explanations into their framework, and apply RexNet to other forms of data, such as audio for abnormal heart murmurs or the vibrations of malfunctioning equipment. This work is one of a body of research being done at the NUS Ubicomp Lab. They will also be presenting other works at CHI 2022 towards usable XAI, including debiasing explanations of corrupted images, as well as improving creativity using AI explanations.
“We live in exciting times to have AI as very capable delegates to many tasks and activities,” says Lim. “But they are currently silent partners (without any explanations), or overly technical ‘geeks’ with jargon speech (prior XAI).”
“Just as we want colleagues who can explain their thought processes relatably, so too should we expect of our AI colleagues,” he adds.
Lim says: “I am thrilled to be able to foster the close partnership between people and AI through human-centered explainable AI.”
Paper: Towards Relatable Explainable AI with the Perceptual Process